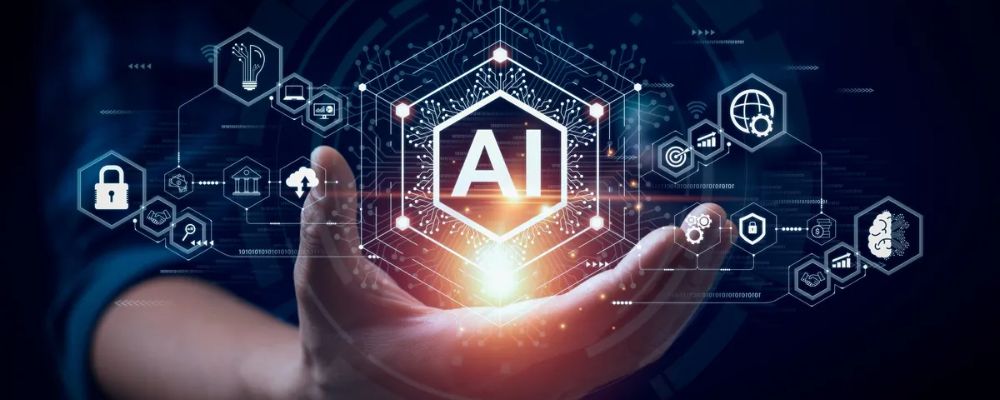
Artificial Intelligence (AI) has transformed numerous aspects of modern life, from streamlining business processes to personalizing user experiences. However, as AI systems become more embedded in decision-making processes, it’s critical to address the issue of AI bias and its associated risks. Algorithmic discrimination, a direct result of AI bias, can lead to significant ethical and practical challenges. This blog will delve into the nature of AI bias, its consequences, and strategies for mitigating these risks to foster fairer AI systems.
What is AI Bias?
AI bias refers to systematic errors or prejudices present in AI algorithms, leading to unfair or inaccurate outcomes. Typically, this bias originates from the data used to train AI models. When the training data is skewed or not representative, the AI system may perpetuate or even exacerbate existing biases.
For instance, biased training data can result in discriminatory practices in hiring, unequal law enforcement, or skewed credit assessments.
Types of AI Bias
Data Bias
Data bias occurs when the dataset used to train the AI model is not representative of the broader population. For example, if a facial recognition system is trained mostly on images of one ethnic group, it might perform poorly on individuals from other ethnicities.
Algorithmic Bias
Even with balanced data, algorithms themselves can introduce bias. This can arise from the design of the algorithm or the methods used to process the data.
Human Bias
The biases of developers and engineers can unintentionally influence AI system design and implementation. These biases may affect data selection, algorithm design, and result interpretation.
The Risks of Algorithmic Discrimination
Ethical Concerns
Algorithmic discrimination can lead to unfair treatment based on characteristics such as race, gender, or age. This raises serious ethical issues regarding equality and justice.
Legal Repercussions
Organizations using biased AI systems may face legal challenges and regulatory scrutiny. With increasing regulations addressing algorithmic discrimination, companies must ensure compliance to avoid potential penalties.
Reputation Damage
Bias in AI can damage an organization’s reputation, leading to a loss of trust and customer loyalty. Companies known for unfair or discriminatory practices may struggle to repair their public image.
Operational Inefficiencies
Discriminatory AI systems can lead to poor decision-making, affecting operational efficiency. For example, biased recruitment algorithms may overlook qualified candidates, resulting in suboptimal hiring decisions.
Strategies for Addressing AI Bias
Diverse Data Collection
To mitigate data bias, ensure that training datasets are comprehensive and representative of all relevant demographic groups. This approach helps improve the fairness of AI outcomes.
Bias Detection Tools
Utilize tools and techniques designed to detect and measure bias in AI systems. Regular audits and evaluations can identify and address biases before they impact decision-making.
Transparent Algorithms
Implement transparency in algorithm design and decision-making processes. Providing clear explanations of AI systems’ functioning can enhance accountability and trust.
Inclusive Development Teams
Build diverse teams of developers and data scientists to bring varied perspectives and reduce the likelihood of inherent biases affecting AI systems.
Ethical Guidelines
Establish and adhere to ethical guidelines and best practices for AI development and deployment. Following these guidelines promotes fairness and equity in AI technologies.
Conclusion
Understanding and addressing AI bias is crucial for developing fair and effective AI systems. By recognizing the risks of algorithmic discrimination and taking proactive measures, businesses and developers can contribute to creating more equitable AI technologies. Embracing diversity, transparency, and ethical practices will help ensure that AI systems serve all individuals fairly and justly.