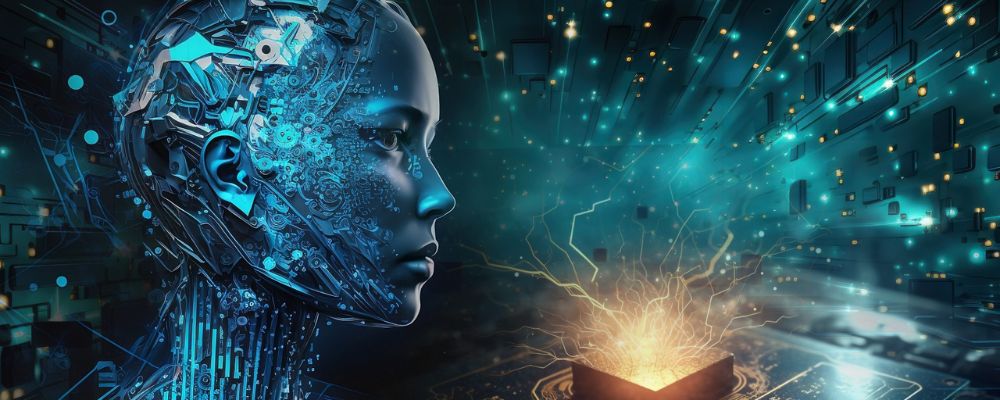
As artificial intelligence (AI) reshapes industries, the need for specialized hardware to efficiently process machine learning (ML) tasks grows. AI hardware, especially AI chips, leads this evolution, offering unmatched computational power, efficiency, and adaptability. This blog explores the future of AI hardware, highlighting the design and development of chips optimized for machine learning.
The Need for AI-Specific Hardware
Traditional central processing units (CPUs) and graphics processing units (GPUs) have supported computing for years. However, as AI workloads become more complex, these general-purpose processors often lack the performance needed for deep learning, natural language processing, and real-time data analysis. This gap has driven the rise of AI-specific chips designed to meet the unique demands of machine learning algorithms.
Key Innovations in AI Chip Design
- Neuromorphic Computing: Neuromorphic chips mimic the human brain’s architecture, enabling faster and more efficient processing of neural networks. These chips handle the parallelism in AI tasks, reducing latency and power consumption. Companies like Intel and IBM are pioneering this technology, which holds promise for AI hardware’s future.
- Tensor Processing Units (TPUs): Google developed TPUs to accelerate machine learning workloads, particularly in training and inference for deep learning models. TPUs offer higher performance per watt than traditional GPUs, making them ideal for large-scale AI applications.
- Field-Programmable Gate Arrays (FPGAs): FPGAs allow developers to customize the chip’s configuration to suit specific tasks, providing flexibility in AI hardware design. This adaptability is crucial as AI models evolve, ensuring that the hardware keeps pace with software advancements.
- Application-Specific Integrated Circuits (ASICs): ASICs are custom-designed chips tailored for specific AI applications, such as autonomous driving or facial recognition. By focusing on a narrow set of tasks, ASICs achieve superior performance and efficiency compared to general-purpose processors.
The Role of AI Hardware in Edge Computing
The shift towards edge computing, where data processing occurs closer to the data source, is another significant trend driving AI hardware innovation. Edge AI chips operate in resource-constrained environments, such as mobile devices or IoT sensors, enabling real-time decision-making without cloud-based processing.
These chips must balance power efficiency with computational capability, often incorporating advanced cooling solutions and energy-efficient architectures to meet the demands of edge AI applications. As the Internet of Things (IoT) expands, developing AI hardware for edge computing becomes increasingly critical.
Challenges in AI Chip Design
Despite the promise of AI hardware, several challenges must be addressed to realize its full potential:
- Thermal Management: Managing the heat generated by powerful AI chips is a significant concern. Efficient cooling solutions are essential to prevent overheating and ensure long-term reliability.
- Energy Efficiency: With the growing emphasis on sustainability, designing AI chips that deliver high performance while minimizing energy consumption is crucial. Chip architecture and materials will play a vital role in achieving this balance.
- Scalability: As AI models grow in size and complexity, scaling AI hardware to meet these demands becomes essential. This includes increasing computational power and optimizing data flow and memory access.
The Future Outlook
The future of AI hardware lies in the continued development of powerful and adaptable chips. As AI models evolve, so must the hardware that powers them. Innovations in neuromorphic computing, TPUs, FPGAs, and ASICs are just the beginning. The next generation of AI chips will likely incorporate advances in quantum computing, bio-inspired architectures, and AI-designed hardware.
In conclusion, designing and developing AI-specific hardware will play a critical role in the growth and success of artificial intelligence. By addressing challenges and seizing opportunities in this field, we can unlock new levels of performance and efficiency in machine learning, paving the way for the next wave of AI-driven innovation.