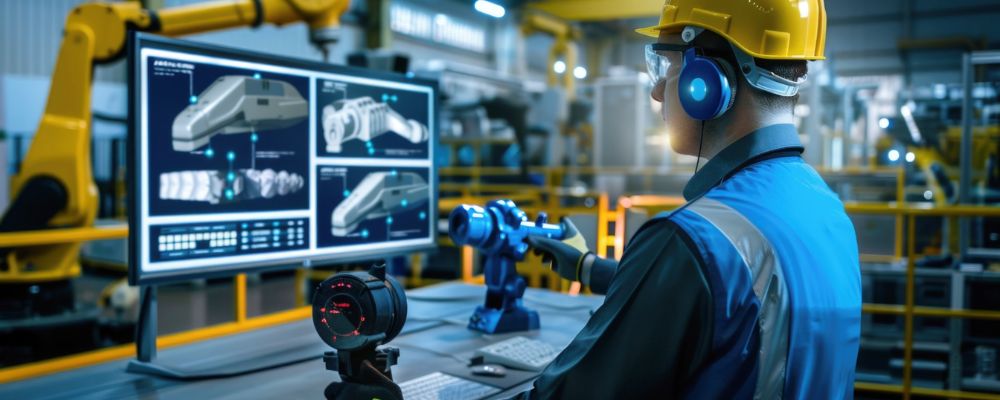
In today’s fast-paced manufacturing industry, one of the most pressing challenges is managing equipment reliability while reducing downtime. Unexpected breakdowns can lead to costly repairs, production delays, and decreased product quality. However, with the advancements in AI-driven predictive maintenance, manufacturers can now stay one step ahead of machine failures, ultimately improving operational efficiency.
Predictive maintenance in manufacturing leverages artificial intelligence (AI), machine learning, and data analytics to forecast equipment failures before they happen. This allows manufacturers to perform maintenance activities only when necessary, instead of relying on scheduled or reactive maintenance strategies. The result is a significant reduction in both maintenance costs and equipment downtime, leading to a more productive and cost-efficient operation.
In this blog, we’ll explore how AI is transforming predictive maintenance in manufacturing, the benefits it offers, and how businesses can implement AI-driven predictive maintenance solutions to optimize their operations.
What is Predictive Maintenance?
Predictive maintenance (PdM) refers to the use of AI, sensors, and data analytics to predict when equipment is likely to fail or require maintenance. This approach is based on real-time data collected from machinery, such as temperature, vibration, sound, and pressure, which is then analyzed using advanced algorithms. By detecting anomalies and predicting failure patterns, manufacturers can take timely action, ensuring machines operate efficiently without unexpected breakdowns.
How Does AI Help in Predicting Maintenance Needs?
AI-driven predictive maintenance relies heavily on machine learning (ML) models and deep learning algorithms. These models can analyze historical data and sensor inputs from machines, detecting patterns that might indicate a potential failure. Through continuous learning, AI systems can improve their predictions over time.
Here’s how AI helps in predicting maintenance needs:
- Data Collection: Sensors attached to machinery collect a wealth of operational data in real-time.
- Data Analysis: AI models analyze this data to identify trends, patterns, and correlations that humans may miss.
- Prediction: Based on this analysis, the AI system predicts when a machine will require maintenance or is likely to fail.
- Actionable Insights: The system generates insights and alerts maintenance teams about potential issues before they occur, allowing for proactive intervention.
Key Benefits of Predictive Maintenance with AI
Implementing AI-driven predictive maintenance in manufacturing offers several key benefits:
1. Reduced Downtime
One of the most significant advantages of predictive maintenance is the reduction in machine downtime. By identifying potential failures before they happen, manufacturers can schedule maintenance during non-production hours, ensuring minimal disruption to operations.
2. Cost Savings
With predictive maintenance, manufacturers only perform maintenance when necessary, rather than adhering to fixed schedules or reacting to failures. This results in significant cost savings, as unnecessary maintenance tasks are eliminated, and expensive emergency repairs are minimized.
3. Improved Equipment Lifespan
Predictive maintenance allows manufacturers to address issues before they escalate into more significant problems. By fixing minor issues early on, the overall lifespan of equipment is extended, and the need for costly replacements is reduced.
4. Enhanced Safety
By detecting potential issues before they cause failures, predictive maintenance helps prevent accidents that could endanger workers or damage equipment. AI systems can also monitor critical parameters such as temperature and pressure to ensure machines are operating within safe limits.
5. Optimized Resource Allocation
Predictive maintenance allows manufacturers to optimize their resource allocation. Instead of scheduling routine maintenance for all machines, resources are directed only to those machines that need attention, improving overall efficiency.
How AI and Machine Learning Enable Predictive Maintenance
AI and machine learning are key enablers of predictive maintenance. By using large datasets and complex algorithms, AI systems can identify hidden patterns and anomalies that might indicate an impending failure. Below are some common machine learning techniques used in predictive maintenance:
1. Supervised Learning
In supervised learning, AI models are trained on labeled data, where the input features and corresponding output (such as failure occurrence) are provided. The model then learns to predict future maintenance needs based on historical data.
2. Unsupervised Learning
Unsupervised learning allows AI models to identify patterns in data without predefined labels. This technique is especially useful when there’s a lack of labeled failure data. The AI system can detect unusual patterns or outliers that might indicate potential issues.
3. Reinforcement Learning
Reinforcement learning involves teaching AI systems to make decisions by rewarding them for taking actions that lead to favorable outcomes. This approach can be applied to optimize maintenance scheduling, ensuring that the system learns the most effective maintenance strategies over time.
Challenges in Implementing AI for Predictive Maintenance
While the benefits of AI-powered predictive maintenance are clear, there are several challenges manufacturers may face when implementing these solutions:
1. Data Quality and Availability
AI systems rely on large volumes of high-quality data to make accurate predictions. If the data collected from machines is inaccurate or incomplete, the AI system’s predictions will be unreliable. Ensuring data consistency and quality is crucial for successful implementation.
2. Integration with Legacy Systems
Many manufacturers still rely on legacy equipment and systems that may not be compatible with modern AI technologies. Integrating predictive maintenance solutions with these legacy systems can be challenging and may require significant investment in upgrading infrastructure.
3. Skilled Workforce
AI and machine learning technologies require a skilled workforce capable of managing, analyzing, and interpreting the data. Manufacturers may need to invest in training their staff or hire AI experts to implement and manage predictive maintenance systems effectively.
4. Cost of Implementation
The initial investment in AI-driven predictive maintenance systems can be significant. However, the long-term savings and benefits often outweigh the initial costs, making it a worthwhile investment for many manufacturers.
Best Practices for Implementing Predictive Maintenance in Manufacturing
To successfully implement AI-powered predictive maintenance in manufacturing, here are some best practices:
1. Start with a Pilot Project
Instead of implementing predictive maintenance across all machines immediately, manufacturers should begin with a small-scale pilot project. This allows for testing and fine-tuning the AI models before scaling up to the entire facility.
2. Invest in High-Quality Sensors
The success of predictive maintenance depends heavily on the quality of the data collected. Manufacturers should invest in high-quality sensors that can monitor various machine parameters in real-time.
3. Ensure Data Integrity
To build reliable AI models, manufacturers must ensure the integrity and accuracy of the data collected. Data cleaning and validation processes should be in place to maintain high-quality datasets.
4. Train Staff for AI Adoption
AI adoption requires a knowledgeable workforce. Manufacturers should invest in training their staff to understand AI and its role in predictive maintenance. Collaboration between data scientists and maintenance teams is crucial for success.
Future Trends in AI for Predictive Maintenance
The future of predictive maintenance looks promising, with AI technology advancing rapidly. Some emerging trends in the field include:
- Edge Computing: With edge computing, data is processed closer to the source, allowing for faster analysis and decision-making in real-time.
- IoT Integration: The Internet of Things (IoT) will play a key role in predictive maintenance by connecting machines and sensors, enabling better data collection and analysis.
- Advanced AI Algorithms: As AI algorithms evolve, they will become even more accurate and capable of predicting maintenance needs with greater precision.
Conclusion
Predicting maintenance needs in manufacturing through AI is revolutionizing the way industrial operations are managed. By utilizing AI and machine learning models, manufacturers can significantly reduce downtime, cut maintenance costs, extend equipment life, and enhance safety. While implementing AI for predictive maintenance comes with its own set of challenges, the benefits it brings to manufacturing operations are immense. As AI technology continues to evolve, the future of predictive maintenance will only become more accurate, efficient, and accessible to manufacturers worldwide.