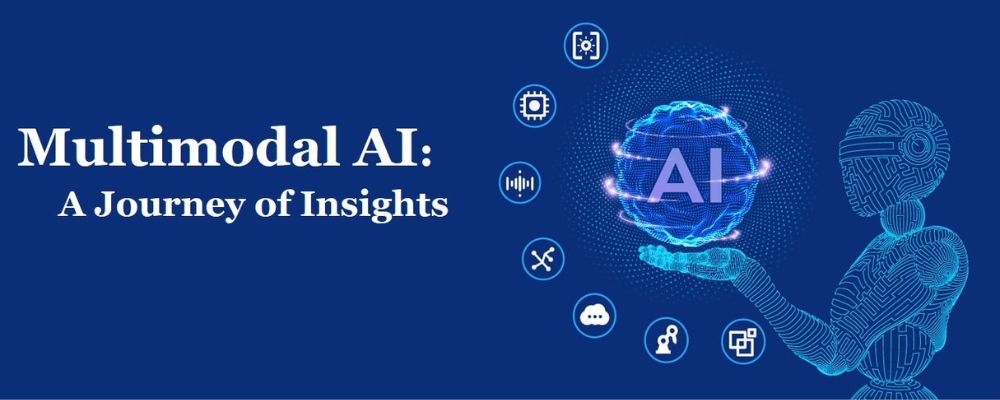
In the rapidly evolving landscape of artificial intelligence (AI), multimodal AI models are emerging as a powerful tool, integrating text, image, and audio analysis to create more comprehensive and sophisticated systems. These models leverage data from multiple modalities, enabling more accurate and nuanced understanding, which is transforming various industries, from healthcare to entertainment. This blog explores the concept of multimodal AI, its benefits, applications, and future trends.
Understanding Multimodal AI
What is Multimodal AI?
Multimodal AI refers to the integration of multiple types of data, such as text, images, and audio, into a single model to enhance its ability to understand and generate complex information. Unlike traditional AI models that focus on a single data type, multimodal AI models can process and analyze diverse data sources simultaneously, leading to more robust and versatile AI systems.
How Does Multimodal AI Work?
Multimodal AI models combine various machine learning techniques, including natural language processing (NLP), computer vision, and audio analysis, to interpret and correlate information from different modalities. These models use deep learning architectures like convolutional neural networks (CNNs) for image analysis, recurrent neural networks (RNNs) for text and audio processing, and transformer models for integrating and understanding multimodal data.
Benefits of Multimodal AI
1. Enhanced Understanding
By integrating multiple data types, multimodal AI models can achieve a deeper and more comprehensive understanding of information. This is particularly useful in scenarios where context from different modalities is essential for accurate interpretation.
2. Improved Accuracy
Multimodal AI models often exhibit higher accuracy compared to unimodal models, as they can cross-reference and validate information from different sources. This reduces the likelihood of errors and enhances the reliability of AI predictions and analyses.
3. Versatility
The ability to process text, images, and audio makes multimodal AI models highly versatile, allowing them to be applied across a wide range of applications and industries. This versatility leads to the development of more innovative and effective solutions.
4. Better User Experience
Multimodal AI can significantly improve user experiences by providing more natural and intuitive interactions. For example, virtual assistants that understand both spoken commands and visual cues can offer more accurate and helpful responses.
Applications of Multimodal AI
1. Healthcare
In healthcare AI, multimodal AI models can integrate patient records, medical images, and spoken symptoms to provide comprehensive diagnostics and personalized treatment plans. For example, combining radiology images with patient history and genetic data can enhance the accuracy of disease detection and treatment recommendations.
2. Autonomous Vehicles
Autonomous vehicles rely on multimodal AI to integrate data from cameras, LIDAR, radar, and audio sensors to navigate and make real-time decisions. This integration enables safer and more efficient autonomous driving by providing a complete understanding of the vehicle’s surroundings.
3. Entertainment
Multimodal AI is revolutionizing the entertainment industry by enabling the creation of more immersive and interactive experiences. For instance, AI models can analyze video content, recognize faces and objects, and generate real-time subtitles or descriptions, enhancing accessibility and user engagement.
4. Customer Service
Multimodal AI models can improve customer service by integrating text-based chatbots with voice recognition and image analysis. This allows for more dynamic and effective customer interactions, where the AI can understand and respond to customer queries through multiple channels.
5. Security and Surveillance
In security and surveillance, multimodal AI models can analyze video feeds, audio signals, and textual data to detect suspicious activities and generate alerts. This comprehensive analysis enhances the accuracy and effectiveness of security systems.
6. Education
Multimodal AI can transform education by providing personalized learning experiences. For example, educational platforms can use AI to analyze student performance across written assignments, spoken presentations, and visual projects, offering tailored feedback and resources to improve learning outcomes.
Future Trends in Multimodal AI
1. Advancements in Deep Learning Architectures
Future developments in deep learning architectures will enhance the capabilities of multimodal AI models. Innovations such as more efficient transformer models and improved neural network architectures will enable even more accurate and robust integration of multimodal data.
2. Real-Time Multimodal Processing
Advances in computing power and algorithms will enable real-time processing of multimodal data, making AI systems more responsive and interactive. This will be particularly beneficial in applications such as autonomous vehicles, virtual assistants, and interactive entertainment.
3. Ethical and Responsible AI
As multimodal AI models become more prevalent, ensuring ethical and responsible use of these technologies will be crucial. This includes addressing issues such as bias, privacy, and transparency to build trust and ensure the fair and equitable application of AI.
4. Integration with Edge Computing
The integration of multimodal AI with edge computing will enable real-time data processing at the source, reducing latency and improving efficiency. This will be particularly important for applications requiring immediate responses, such as autonomous driving and real-time surveillance.
5. Enhanced Human-AI Collaboration
Future multimodal AI systems will enhance human-AI collaboration by providing more intuitive and natural interactions. For example, AI-powered tools that understand and respond to multiple forms of human communication will enable more effective collaboration in various fields, from creative industries to scientific research.
Conclusion
Multimodal AI models represent a significant advancement in the field of artificial intelligence, offering enhanced understanding, improved accuracy, and greater versatility by integrating text, image, and audio analysis. With applications spanning healthcare, autonomous vehicles, entertainment, customer service, security, and education, the potential of multimodal AI is vast. As technology continues to evolve, the future of multimodal AI looks promising, with exciting opportunities for innovation and growth.
By staying informed about the latest developments and embracing multimodal AI, we can unlock new possibilities and create more sophisticated, accurate, and versatile AI systems that benefit society as a whole.