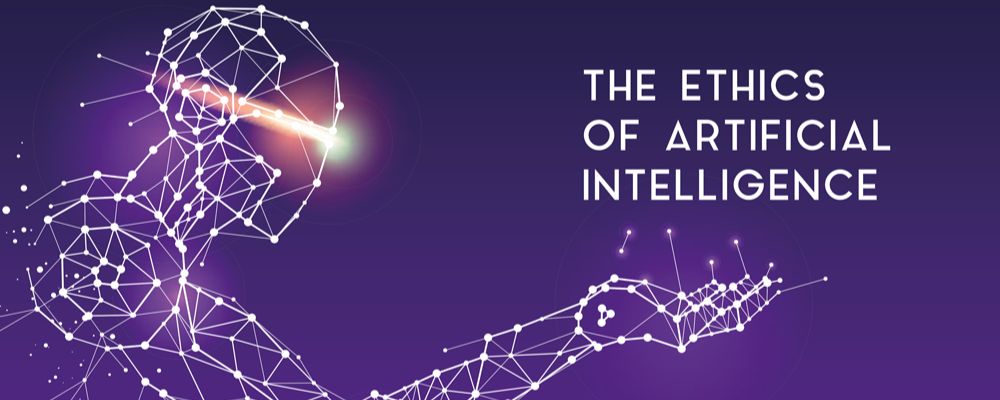
As Artificial Intelligence (AI) and machine learning (ML) systems become increasingly integrated into various aspects of society, concerns about bias and fairness have come to the forefront. Ensuring that these technologies operate ethically is crucial for maintaining public trust and achieving equitable outcomes. This blog will explore the sources of bias in AI, the impact of bias on society, and strategies to promote fairness and ethical AI practices.
Understanding Bias in AI
Bias in AI systems often arises from the data used to train these models. If the training data contains historical biases or is unrepresentative of the population, the AI system can learn and perpetuate these biases. Bias can manifest in various forms, including:
- Selection Bias: Occurs when the data used to train an AI model is not representative of the target population.
- Label Bias: Happens when the labels in the training data are influenced by human prejudices or errors.
- Measurement Bias: Results from inaccuracies in the data collection process.
Impact of AI Bias on Society
The presence of bias in AI systems can have significant negative consequences, including:
- Discrimination: Biased AI systems can disproportionately impact certain groups, leading to unfair treatment in areas such as hiring, lending, and law enforcement.
- Loss of Trust: If AI systems are perceived as biased or unfair, public trust in these technologies can erode.
- Inequity: Bias can exacerbate existing inequalities, making it harder for disadvantaged groups to access opportunities and resources.
Strategies for Addressing Bias and Promoting Fairness
Ensuring fairness and mitigating bias in AI systems requires a multifaceted approach. Here are some key strategies:
1. Diverse and Representative Data
Using diverse and representative datasets is fundamental to reducing bias in AI systems. Ensuring that the training data reflects the diversity of the population helps create more equitable models. For more on data diversity, check out Nature’s insights on AI training data.
2. Bias Detection and Mitigation Techniques
Implementing techniques to detect and mitigate bias during the model development process is crucial. This includes:
- Pre-processing: Modifying the training data to reduce bias before training the model.
- In-processing: Adjusting the learning algorithm to mitigate bias during training.
- Post-processing: Modifying the model’s predictions to ensure fairness after training.
3. Ethical Guidelines and Regulations
Developing and adhering to ethical guidelines and regulations can help ensure that AI systems are designed and deployed responsibly. Organizations should establish clear policies for ethical AI use and comply with relevant regulations, such as the General Data Protection Regulation (GDPR) and the Health Insurance Portability and Accountability Act (HIPAA).
4. Transparency and Explainability
Enhancing the transparency and explainability of AI systems can help build trust and allow for better scrutiny of potential biases. Providing clear explanations of how AI models make decisions enables stakeholders to understand and address any underlying biases.
5. Ongoing Monitoring and Evaluation
Continuous monitoring and evaluation of AI systems are essential to identify and rectify biases that may emerge over time. Regular audits and assessments can help ensure that AI models remain fair and equitable.
Case Studies Highlighting Bias in AI
To illustrate the real-world implications of bias in AI, let’s look at some notable case studies:
1. Facial Recognition Technology
Facial recognition systems have been criticized for exhibiting significant bias, particularly against people of color and women. Studies have shown that these systems often misidentify individuals from these groups at higher rates compared to white males. This bias can lead to wrongful accusations and reinforce existing societal inequalities.
2. Predictive Policing
Predictive policing algorithms, designed to forecast where crimes are likely to occur, have been found to disproportionately target minority communities. This can result in over-policing and increased scrutiny of these communities, further perpetuating cycles of disadvantage and mistrust in law enforcement.
3. Hiring Algorithms
Several companies have used AI-driven hiring tools to screen job applicants. However, these systems have sometimes shown bias against certain demographic groups, particularly women and minorities. This occurs when the training data reflects historical biases in hiring practices, leading the AI to favor candidates that resemble those who were previously hired.
Promoting Fairness in AI Development
Addressing bias in AI is not just a technical challenge; it also requires a commitment to ethical principles and societal values. Here are some steps that can be taken to promote fairness in AI development:
1. Inclusive Design Practices
Involving diverse teams in the design and development of AI systems can help ensure that different perspectives are considered, reducing the likelihood of bias. This includes not only diversity in terms of gender, race, and ethnicity but also in terms of expertise and experience.
2. Public and Stakeholder Engagement
Engaging with the public and stakeholders can provide valuable insights into the potential impacts of AI systems and help identify areas of concern. This can be done through public consultations, focus groups, and collaborations with advocacy organizations.
3. Ethical Review Boards
Establishing ethical review boards can help oversee AI development projects and ensure that ethical considerations are incorporated into every stage of the process. These boards can provide guidance on issues such as data privacy, consent, and fairness.
4. Research and Innovation
Ongoing research into bias mitigation techniques and fairness measures is crucial for advancing the field of ethical AI. This includes developing new algorithms that are less prone to bias and creating tools to assess and address bias in existing systems.
Conclusion
As AI continues to evolve and become more integrated into our daily lives, addressing bias and promoting fairness in machine learning is paramount. By understanding the sources of bias, recognizing its impact on society, and implementing strategies to mitigate it, we can work towards creating AI systems that are not only powerful and efficient but also just and equitable.
The future of ethical AI depends on our collective efforts to prioritize fairness and transparency. By fostering a culture of ethical AI development and adhering to best practices, we can harness the potential of AI to benefit all of society, ensuring that no group is left behind.